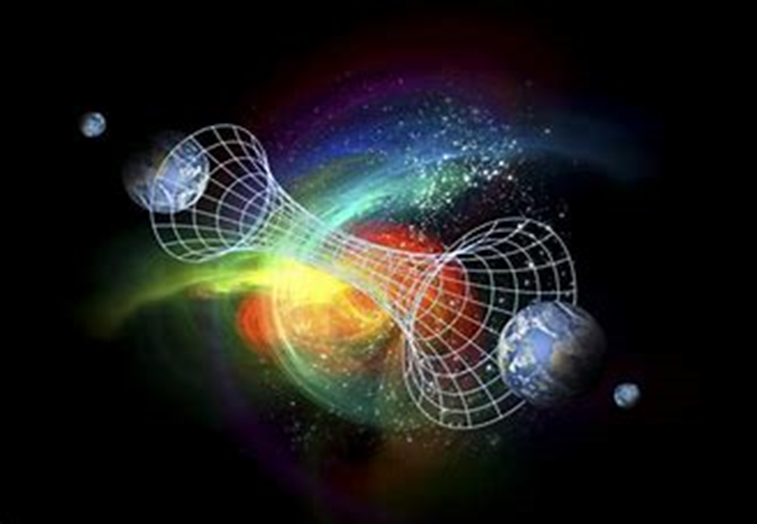
The primary objective of this course is to train students in causal inference analysis, i.e., techniques for identifying the cause-and-effect relationship between events. This is typically done by comparing the results of an intervention/policy/shock on a group of people (called treated) with a control group that does not receive the intervention. The goal of causal inference is to determine whether the intervention had a significant effect on the outcome, and to quantify the strength of the relationship between the intervention and the outcome.
Two techniques are going to be studied here:
- Difference-in-differences
- Regression Discontinuity Design
In each case, examples applied to energy and environmental issues are going to illustrate the scientific usefulness of these techniques.
This course will help students to develop a number of important skills, including:
Understanding the concept of cause and effect and how it applies to the study of real-world phenomena.
Familiarity with the different methods used to identify and estimate causal effects, including experimental and observational designs.
The ability to use statistical software to analyze data and test hypotheses about causal relationships (STATA, R, Python).
The ability to interpret and communicate the results of causal inference analyses in a clear and concise manner.
The ability to critically evaluate the assumptions and limitations of different causal inference methods and apply them appropriately to different research questions.
Overall, a course on causal inference can help students develop the skills and knowledge needed to conduct rigorous, evidence-based research that can inform decision-making and policy-making in the field of energy and environmental economics (and beyond).
- Irakaslea: Fabien Candau